Revolutionize Product Recommendations with Amazon Titan Multimodal Embeddings
The rise of contextual and semantic search in ecommerce is changing the game. Discover how the Amazon Titan Multimodal Embeddings model is transforming product recommendations.

The Power of Generative AI in Product Recommendations
As the world of ecommerce continues to evolve, the importance of accurate and intuitive product search cannot be overstated. With the advent of generative AI-powered search engines and recommendation systems, businesses are now able to offer their customers a seamless and personalized shopping experience like never before.
One key player in this revolution is the Amazon OpenSearch Service, which now supports the cosine similarity metric for k-NN indexes. By harnessing the power of cosine similarity, businesses can ensure that their search results are not just relevant but highly accurate, making it easier for customers to find exactly what they are looking for.
Building a Contextual Text and Image Search Engine
At the forefront of this transformation is the Amazon Titan Multimodal Embeddings model, available in Amazon Bedrock. This groundbreaking model is designed to learn joint representations of different modalities such as text, images, and audio, providing a comprehensive understanding of user queries.
- Amazon Titan Multimodal Embeddings model - This powerful foundation model generates embeddings for product images, enabling businesses to store and retrieve these embeddings in a vector database. By leveraging this model, businesses can enhance their understanding of customer queries and provide more meaningful search results.
- Amazon OpenSearch Serverless - This on-demand serverless configuration serves as the vector database for storing embeddings generated by the Amazon Titan Multimodal Embeddings model. By using OpenSearch Serverless, businesses can efficiently manage and query their embeddings to deliver personalized search results to users.
- Amazon SageMaker Studio - An integrated development environment for machine learning practitioners, SageMaker Studio provides a seamless platform for building, training, and deploying ML models. With SageMaker Studio, businesses can streamline their ML development process and drive innovation in their product recommendations.
The Solution Design
The solution design consists of two main components: data indexing and contextual search. During the data indexing phase, product images are processed to generate embeddings, which are then stored in the vector data store. This preparation step ensures that the system is ready to deliver accurate search results to users.
In the contextual search phase, user queries in the form of text or images are converted into embeddings, and a similarity search is performed on the vector database. By matching these embeddings to stored embeddings, the system can retrieve and display relevant product recommendations to users.
Implementing the Solution
To implement this innovative solution, businesses must have an AWS account and familiarity with foundational models, Amazon Bedrock, Amazon SageMaker, and OpenSearch Service. Additionally, enabling access to the Amazon Titan Multimodal Embeddings model in Amazon Bedrock is essential for leveraging its capabilities in building a contextual search engine.
By following the steps outlined in the provided resources, businesses can set up the solution seamlessly and begin harnessing the power of generative AI in transforming their product recommendations. From data indexing to real-time multimodal search, each step is crucial in ensuring a smooth and accurate search experience for users.
Leveraging Amazon Titan Multimodal Embeddings for Enhanced Search Experiences
By utilizing the Amazon Titan Multimodal Embeddings model in Amazon Bedrock, businesses can unlock a new level of accuracy and relevance in their product recommendations. The ability to understand user queries across different modalities such as text and images enables businesses to provide contextually relevant search results to their customers.
Whether it's enhancing multimodal search, recommendation systems, or personalization experiences, the Amazon Titan Multimodal Embeddings model offers endless possibilities for businesses looking to revolutionize their ecommerce strategies. With its availability in key AWS Regions and comprehensive documentation, getting started with this powerful model has never been easier.
Start building your next-generation product recommendation engine with Amazon Titan Multimodal Embeddings in Amazon Bedrock today and stay ahead of the curve in the world of ecommerce!
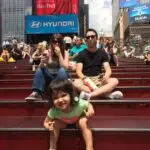
Hey, I'm Saadat Qureshi, your guide through the exciting worlds of education and technology. Originally from Karachi and a proud alum of the University of Birmingham, I'm now back in Karachi, Pakistan, exploring the intersection of learning and tech. Stick around for my fresh takes on the digital revolution! Connect With Me